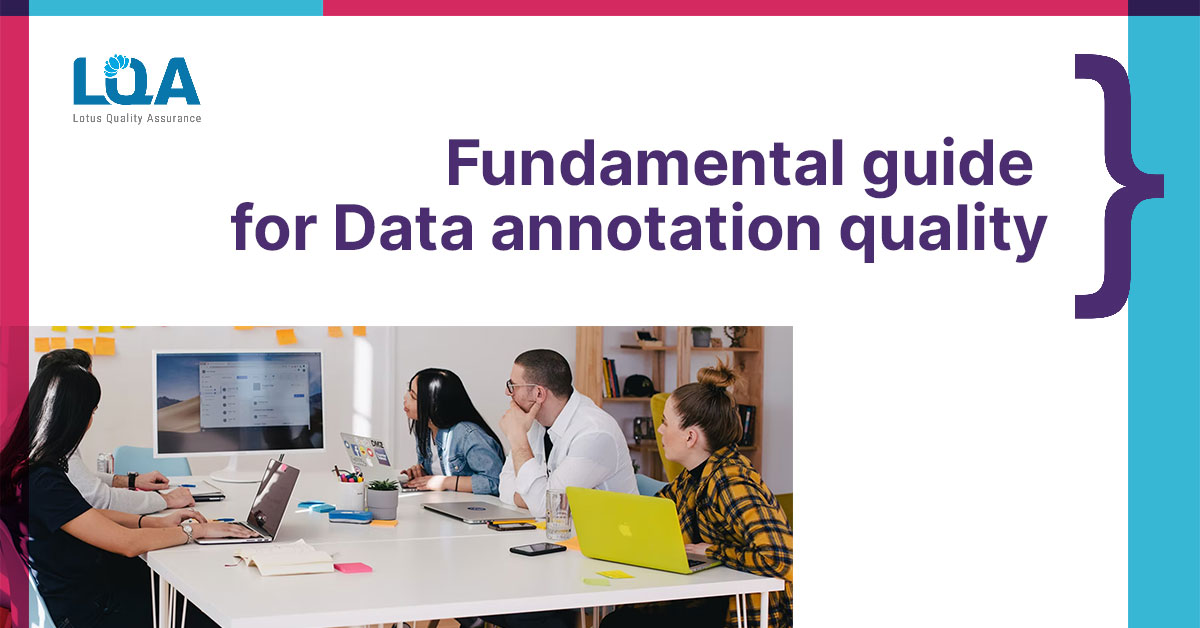
The matter of Data Labeling Quality has been a major topic of concern in AI/ML communities. Perhaps the most common “principle” that you might come across solving this puzzle is “Garbage in, garbage out”.
By saying this, we want to emphasize the fundamental law with training data for artificial intelligence and machine learning development projects. Poor-quality training datasets fed to the AI/ML model can lead to numerous errors in operation.
For example, training data for autonomous vehicles is the deciding factor for whether the vehicles can function on the roads. Provided with poor-quality training data, the AI model can easily mistake humans for an object or the other way round. Either way, the poor training datasets can result in high risks of accidents, which is the last thing that autonomous vehicle manufacturers would want in their projects.
For high-quality training data, we need to involve data labeling quality assurance in the data processing procedure. At Lotus Group and Lotus QA, we take the three following actions to ensure high-quality training datasets. Take a look at this fundamental guide to provide your AI/ML model with the best training data.
Don’t know where to start in AI data processing? Check out our Data Annotation Guide.
1. Clarify requirements to optimize data labeling quality
The precision of annotations
High data labeling quality doesn’t simply mean the most carefully annotated data or the training data of the highest quality. For strategic data annotation projects, we need to clarify the requirements of the training datasets. The questions that annotation team leaders should answer are how high-quality the data needs to be, the acceptable precision of data annotation, and how detailed the output should be.
As a vendor of data annotation quality, one thing that we always ask our clients is the requirements. “How tedious do you want us to work with the datasets?”, “How would you want the precision of our annotations?”. By answering these questions, you will have ahead of you a benchmark for your entire projects later on.
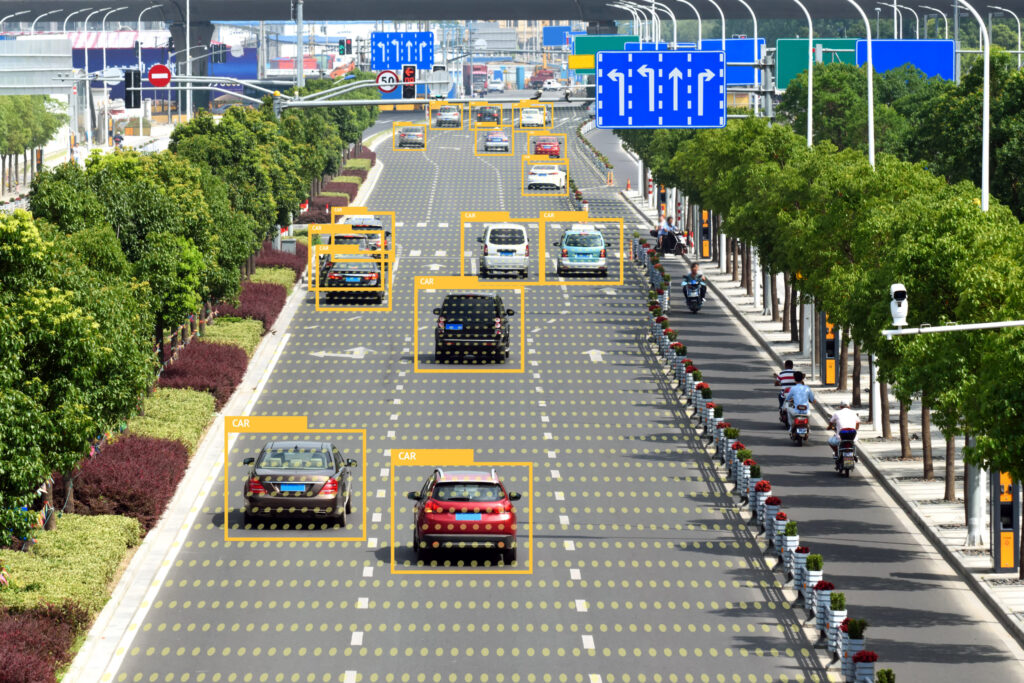
How to ensure data labeling quality
Skillful levels of the annotators
Keep in mind that the implementations of Artificial Intelligence and Machine Learning are very broad. Besides the common applications in autonomous vehicles and transportation, AI and ML made their debut in healthcare and medical, agriculture, fashion, etc. For each and every industry, there are hundreds of different projects, working on different kinds of objects, hence different skills and knowledge are required to ensure data annotation quality.
Take road annotation vs. medical data annotation for example.
- For roads annotation, the work is quite straightforward, and you only need annotators who are capable of common knowledge to do the work. For this annotation project, the number of datasets that need annotating can add up to millions of videos or pictures, and the annotators have to keep the productivity high in an acceptable level of quality.
- Medical data requires annotators who work in the medical field with particular knowledge. For the case of diabetic retinopathy, trained doctors are asked to grade the severity of diabetic retinopathy from photographs so that deep learning can be applied in this particular field.
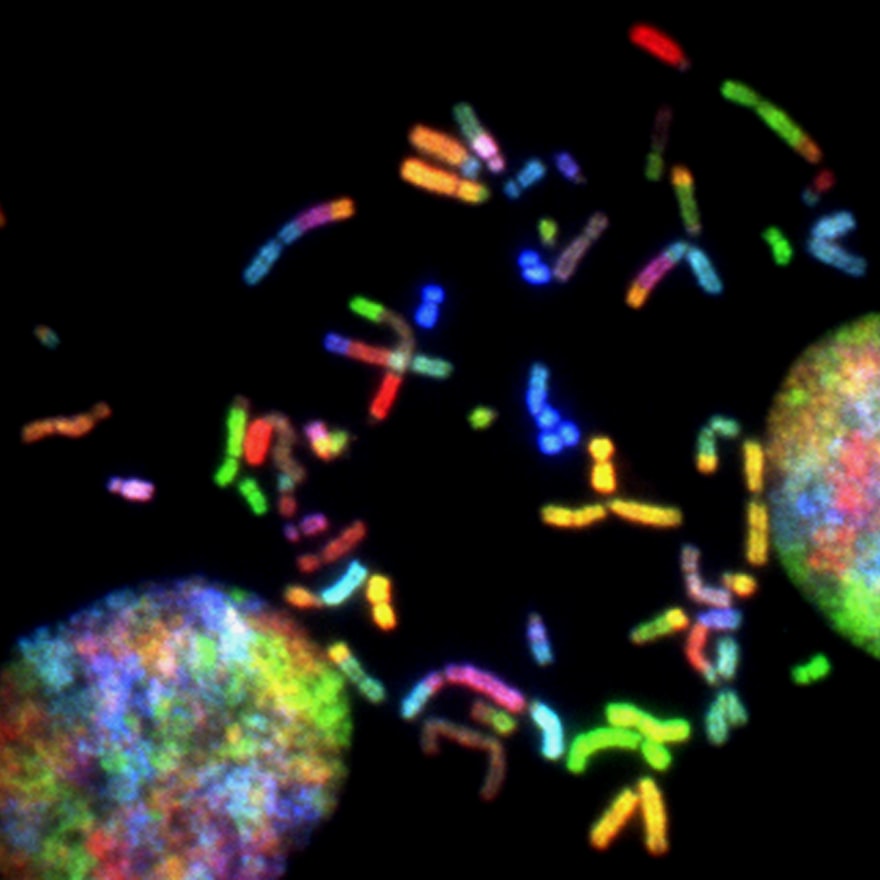
Data labeling quality – With medical use
Even for well-trained doctors, not all of their annotations agree with one another. To have a consistent outcome, one annotation team might have to annotate each file multiple times to eventually come to a correlation.
It is a matter of how complicated the given data is and how detailed the clients want the data output to be. Once these things are clarified, the team leader can work on the allocation of resources for the required outcomes. Metrics and the relevant Quality Assurance process will be defined after this.
Example of an ideal output
We also encourage the clients to provide example sets to act as the “benchmark” for every dataset to be annotated. This is the most straightforward technique for the quality assurance of data annotation that one might employ. With the example of the perfectly annotated data, your annotators now are trained and presented to the baseline of their work.
With the benchmark as the ideal outcome, you can calculate agreement metrics to evaluate each annotator’s accuracy and performance. In case of uncertainty in both the annotation and review process, the QA staff can work with these sample datasets to define which are qualified and which are not.
2. Multi-layered QA process
The QA process in data labeling projects varies within different companies. At LQA, we adhere to the international standardized quality assurance process. The predetermined preferences will always be clarified right at the beginning of the project. These preferences will be compiled into one “benchmark” which will, later on, act as the “golden standard” of every label and annotation.
The steps of this multi-layered QA process are: Self-check, Cross-check, and Manager-check.
Self-check
In this step, annotators are asked to do the review on their own work. With self-assessment, annotators now have the time to look back at the data annotation tool, annotation, and labeling from the start of the project.
Normally, annotators have to work under great pressure in terms of time and workload, which can possibly lead to potential deviations in their work. The quality assurance starting with the self-check step will be the time for annotators to slow down and take a thorough look at how they’ve done. By acknowledging the mistakes and possible deviations, annotators can fix them themselves and avoid any of those in the future.
Cross-check
In data science in general and data annotation in particular, you might have heard about the term “bias”. Annotation bias refers to the situation in which annotators have their own habit to label the data, which can lead to biased opinions upon the provided data. In some cases, annotator bias can influence the model performance. For a more robust AI and ML model, we have to take some effective measures to eliminate the biased annotations, and one simple way to do this is to cross-check.
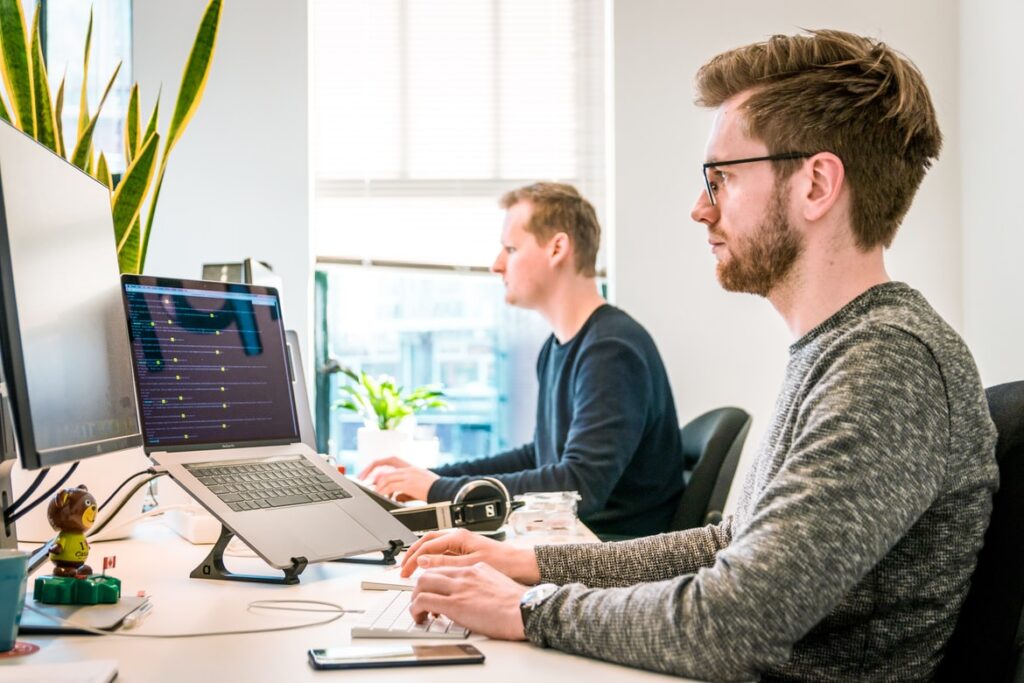
Data Labeling quality – Cross-check
By carrying out cross-checking in your annotation process, the whole work is viewed differently, hence the annotators can identify the mistakes and errors in their colleagues’ work. Again, with this different view, the reviewer can point out the biased annotations and the team leader can take further actions. They can rework or give another round of assessment to see whether the annotations are really biased.
Manager’s review
An annotation project manager is usually responsible for the day-to-day oversight of the annotation project. Their main tasks include selecting/managing the workforce and ensuring data quality and consistency.
The manager will be the one that receives the data sampling from clients and work on the required metrics and carries out training for the annotators. Once the cross-checking is done, the manager can randomly check the output to see whether they adhere to the clients’ requirements.
Prior to all these checks, the annotation project manager also has to draw a “benchmark line” for quality assurance. To ensure annotation consistency and accuracy, any work that is under the predefined quality must be reworked.
3. Quality Assurance staff involvement
Data labeling quality control cannot rely only on the annotation team. In fact, the involvement of professional and experienced quality assurance staff is a must. To ensure the highest quality of your annotation work, a team of quality assurance staff is a must. They will work as an independent department, outside of the annotation team, and not under the management of the annotation project manager.
The ideal percentage of quality staff over the entire number of data annotation staff doesn’t go beyond 10%. The QA staff cannot and will not review every single annotated data in your project. In fact, they will randomly take out datasets and once again, review the annotations.
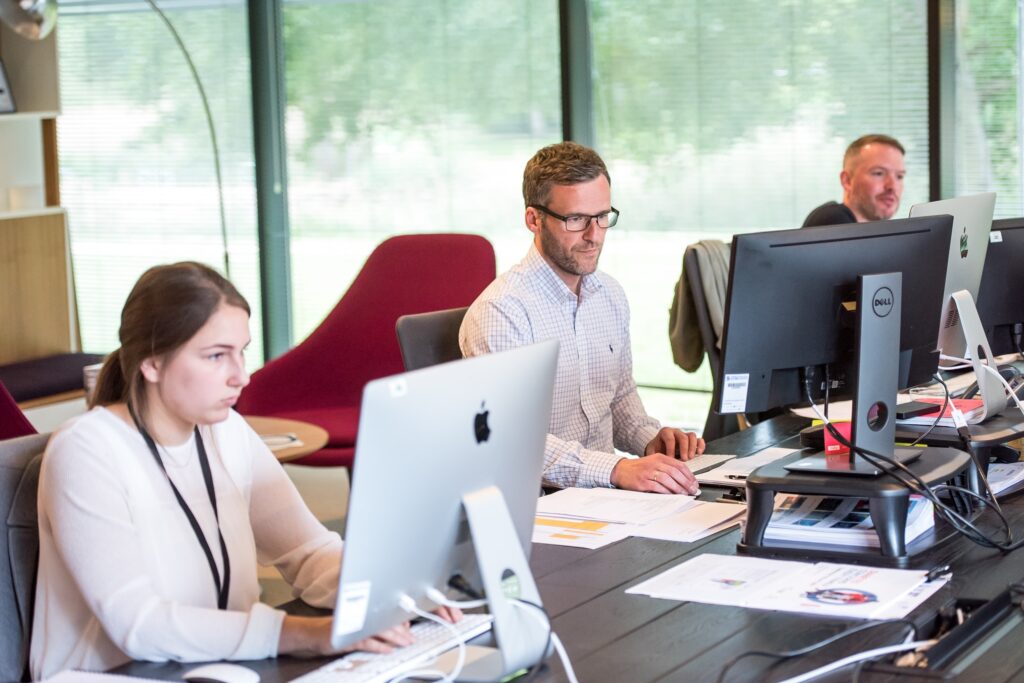
Data Labeling quality – Quality Assurance
These QA staffs are well-trained with the data sample and will have their metrics to evaluate the quality of the annotated data. These metrics must be agreed upon between the QA team leader and the annotation project manager beforehand.
In addition to the three-step of review of self-check, cross-check and manager’s review, the involvement of QA staff in your annotation projects will sure adhere your data output to the predefined benchmark, which eventually ensures the highest level possible for your training data.
Want to hear more from professionals to enhance your data labeling quality? Contact LQA for more information:
- Website: https://www.lotus-qa.com/
- Tel: (+84) 24-6660-7474
- Fanpage: https://www.facebook.com/LotusQualityAssurance