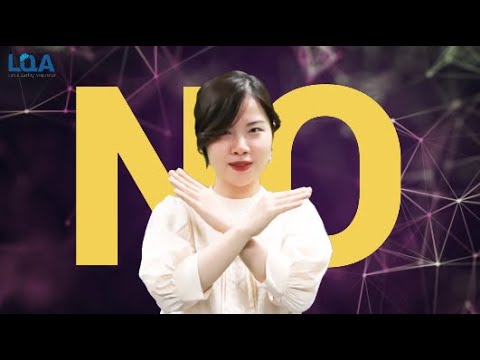
This is the first video of the series 5 Essentials of AI Training Data Labeling work. Ngoc will talk about data quality and its determinants.
You can watch our video here, or read the transcription below. Turn on subtitles for English, Japanese, Korean and Vietnamese
Hello everyone, my name is Bich Ngoc from Sales Department of Lotus Quality Assurance. You can also call me Hachi.
Welcome to LQA channel. Our channel is aimed at sharing information about testing and data annotation for AI development. If you want to see more helpful videos from our channel, please like and subcribe to our channel.
You are…
- Dealing with massive amounts of data you want to use for machine learning?
- Doing most of the work in-house but now you want your team to focus on more strategic initiative?
- Thinking about outsourcing the data annotation work but still have a lot of concerns?
These video series are totally for you.
With 5 videos in the series, we will take you through the essential elements of successfully outsourcing this vital but time consuming work.
Our sharing is not only from the perspective of a data labelling service provider but also a quality assurance company. So I hope you guys will find it fresh and helpful.
- Data Quality
- Scale – What happens when my data labeling volume increases
- Tools – Do I need a tooling platform for data labeling
- Cost
- Security – How will my data be protected
Today I will introduce you to one aspect you have to consider when you prepare a data set for your AI: DATA QUALITY.
What is Data Quality?
First of all, let’s get to know what Data Quality is.
Simply put, data quality is an assessment whether the given data is fit for purpose.
Why is there even a question of quality when it comes to data for AI?
Isn’t having access to huge amounts of data enough?
The answer is no.
Not every kind of data, and not every data source, is useful or of sufficiently high quality for the machine learning algorithms that power artificial intelligence development – no matter the ultimate purpose of that AI application.
To be more specific, the quality of data is determined by accuracy, consistency, completeness, timeliness and integrity.
- Accuracy: It measures how reliable a dataset is by comparing it against a known, trustworthy reference data set.
- Consistency: Data is consistent when the same data located in different storage areas can be considered equivalent.
- Completeness: the data should not have missing values or miss data records.
- Timeliness: the data should be up to date.
- Integrity: High-integrity data conforms to the syntax (format, type, range) of its definition provided by e.g. a data model
Why is data quality important?
For example, if you train a computer vision system for autonomous vehicles with images of mislabelled road lane lines, the results could be disastrous.
In order to develop accurate algorithms, you will need high-quality training data labelled by skilled annotators.
3 Workforce Traits that Affect Quality in Data Labelling
In our years of experience providing managed data labelling teams for start-up to enterprise companies, we’ve learned three workforce traits affect data labelling quality for machine learning projects: knowledge and context, agility and communication.
Knowledge and context
Firstly, for highest quality data, labelers should know key details about the industry you serve and how their work relates to the problem you are solving.
For example, people labeling tomato images will pay more attention to the size, color and the condition of each tomato if they know the data they are labeling will be used to develop AI system supporting tomato harvest.
Agility
Secondly, your data labeling team should have the flexibility to incorporate changes that adjust to your end users’ needs, changes in your product, or the addition of new products.
A flexible data labeling team can react to changes in data volume, task complexity, and task duration.
Communication
Last but not least, you need data labelers to respond quickly and make changes in your workflow, based on what you’re learning in the model testing and validation phase.
To do that kind of agile work, you need direct communication with your labeling team.
To conclude, high-quality training data is necessary for a successful AI initiative.
Before you begin to launch your AI initiative, pay attention to your data quality and develop data quality assurance practices to realize the best return on your investment.
You can watch our next video on Scaling Data Annotation, or other videos in the series.
Also, try out our series on Data Annotation Tools and visit our Youtube Channel
Interested in our Annotation service?