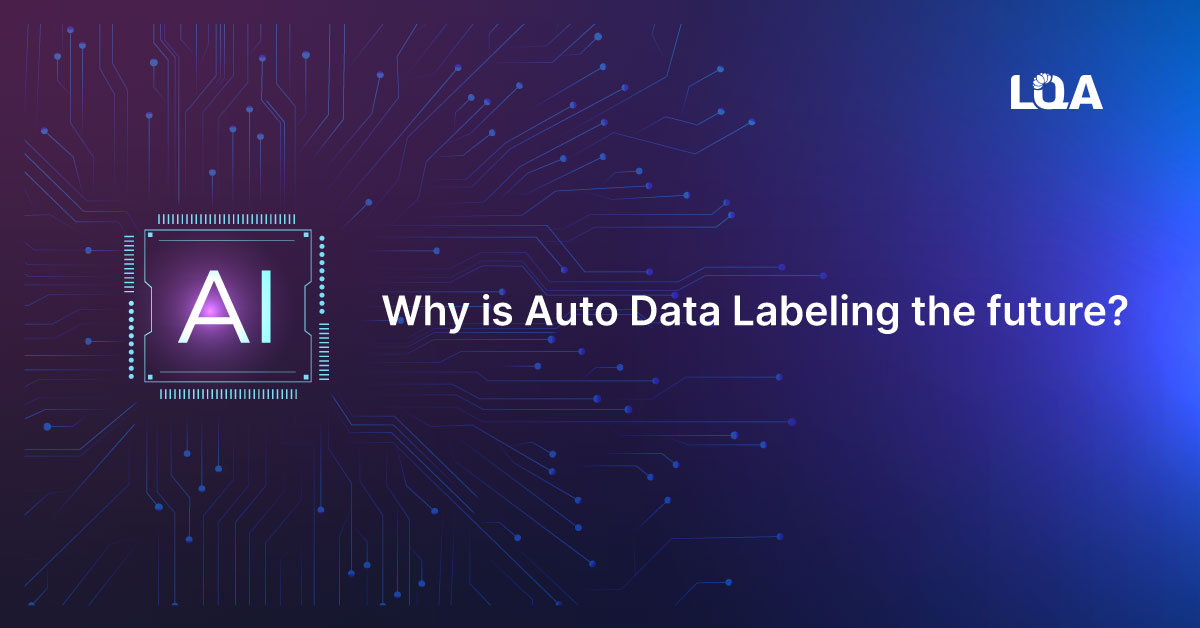
Automated Data Labeling is a new feature that is currently being constantly mentioned among Data annotation trends, and some even deem it the solution for the time-consuming and resource-consuming casual manual annotation.
As the Manual Data Labeling – aka Manual Data Annotation takes hours to annotate one dataset, the Automated data labeling technology now proposes a simpler, faster and more advanced way of processing data, through the use of AI itself.
How we normally handle dataset
The most common and simplest approach to data labeling is, of course, a fully manual one. A human user is presented with a series of raw, unlabeled data (such as images or videos), and is tasked with labeling it according to a set of rules.
For example, when processing image data for machine learning, the most common types of annotations are classification tags, bounding boxes, polygon segmentation, and key points.
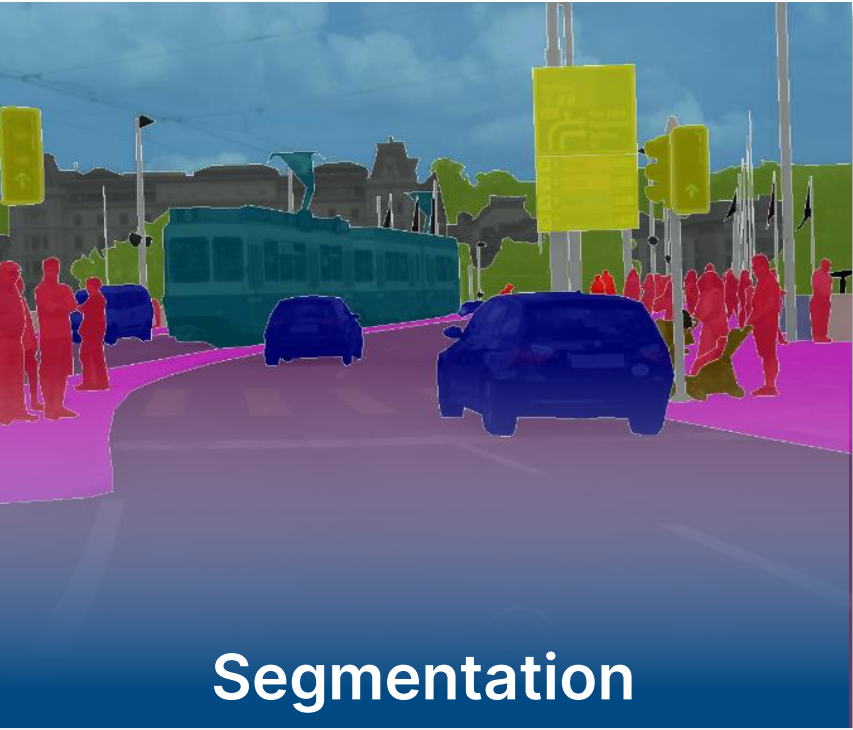
Automated Data Labeling – Segmentation in Data Labeling
Classification tags, which are the easiest and cheapest annotation, may take as little as a few seconds whereas fine-grained polygon segmentation could take a few minutes per each instance of objects.
In order to calculate the impact of AI automation on data labeling times, let’s assume that it takes a user 10 seconds to draw a bounding box around an object, and select the object class from a given list.
In this case, provided with a typical dataset with 100,000 images and 5 objects per image, annotators would have to spend 1,500 man-hours to complete the annotation process. This eventually would cost approximately $10,000 just for data labeling.
The price of $10.000 is only for data labeling. For annotation project managers, AI data processing takes more than that. To ensure the high quality of the training data, they are compelled to add other layers of quality control and quality assurance. This helps manually verify and review each piece of labeled data, but it would be very costly. Moreover, the quality control and quality assurance staff must be trained of the sample output so that they understand what is required in the outcome of the annotation projects, thereby increasing the labeling costs by about 10%.
Some annotation project managers might choose consensus-based quality control. By implementing this method, the whole annotation project goes through multiple annotations. The same piece of data is annotated multiple times, and the results are consolidated and compared for quality control purposes. With this method, the amount of time and money is proportional to the number of annotators working on the same task. Simply put, if you had three users label the same image three times, you would have to pay for all 3 annotations.
All this is to emphasize that, the two most expensive steps in data labeling are:
- The data labeling itself
- Reviewing and verifying it for quality control.
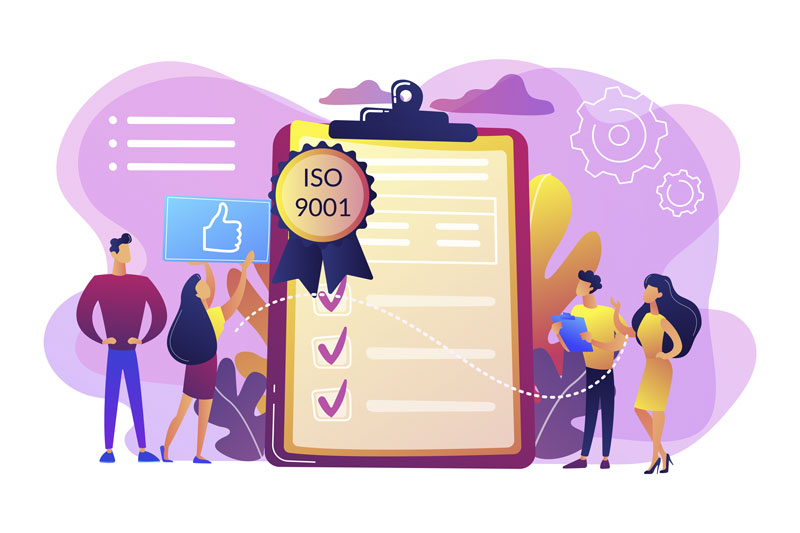
Automated Data Labeling – Emphasis on Quality Control
Looking at all the huge costs that it would take in an annotation project, many business leaders have turned into a less time-consuming and tedious solution, which is the auto annotation tool technology.
Thankfully, with the latest technologies in artificial intelligence and machine learning, automated data labeling, or auto annotation, is usable now. However, to create an effective and well-rounded auto annotation tool now, it even requires more training data and human input for correcting errors induced by the AI. Therefore, anyone has the naive attempt to entirely apply auto annotation tools, they have to be cognizant of the truth that the tools are not the one-size-fits-all solution.
The advantages of Automated Data Labeling
Automated data labeling is quite a new term in the field, but the technology advancement implementing and making it happen is developing with high speed, shown in the large number of tools on the market now. So what are auto data labeling and its benefits?
What’s automated data labeling?
Automatic labeling is a feature found in data annotation tools that apply artificial intelligence (AI) to enrich, annotate, or label a dataset. Tools with this feature augment the work of humans in the loop to save time and money on data labeling for machine learning.
Most tools allow you to load pre-annotated data into the tool. More advanced tools, which are evolving into platforms (e.g., tool plus Software Development Kit or SDK), allow you to leverage AI or bring your own algorithm to the tool to improve the data enrichment process by auto labeling data.
Other tools offer prediction models that suggest annotations so workers can validate them. Some features leverage embedded neural networks that can learn from every annotation made. All of these features can save time and resources for machine learning teams and will have a profound effect on data annotation workflows.
Outstanding benefits of automated data labeling
When working with organizations using tools to annotate images for machine learning, we find two optimal ways to apply auto labeling in data annotation workflow:
- Pre-annotate some or all of your dataset. Workers come behind the automation to review, correct, and complete the annotations. Automation cannot annotate everything; there will be exceptions and edge cases. It’s also far from perfect, so you must plan for people to make reviews and corrections as necessary.
- Reduce the amount of work sent to people. An auto-labeling model can assign a confidence level based on the use case, task difficulty, and other factors. It enriches the dataset with annotations, and sends annotations with lower confidence scores to a person for review or correction.
We’ve run time experiments, with one team using tools that have an automation feature versus another team that is manually annotating the same data. In some cases, we’ve seen auto labeling provide low-quality results which increase the amount of time required per annotation task. Other times, it has provided a helpful starting point and reduced task time.
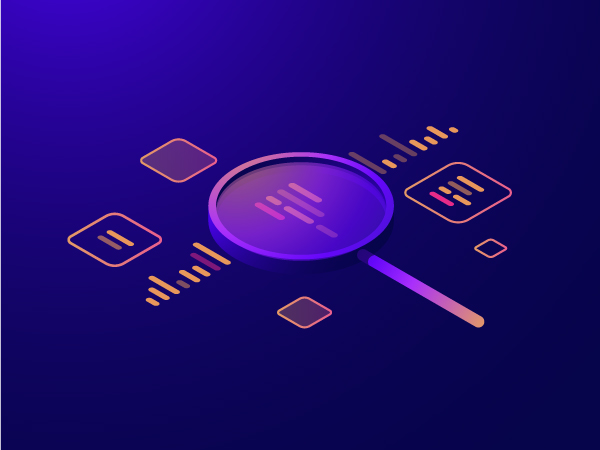
Automatic Data Labeling- Metadata
In one image annotation experiment, auto labeling combined with human-powered review and improvements was 10% faster than the 100% manual labeling process. That time savings increased from 40% to 50% faster as the automation learned over time.
It also had a more than the five-pixel margin of error for vehicles and missed the objects that were farthest from the camera. As you can see in the image, an auto-labeling feature tagged a garbage bin as a person. It’s important to keep in mind that pre-annotation predictions are based on existing models and any misses in the auto labeling reflect the accuracy of those models.
Data annotation tools can include automation, also called auto labeling, such as Labelbox and Tagtog, which uses artificial intelligence to label data, and workers can confirm or correct those labels, saving time in the process.
While auto labeling is not perfect, it can provide a helpful starting point and reduce task time for data labelers.
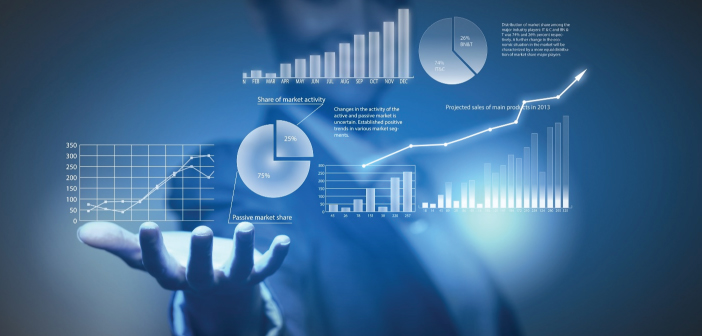
Auto Data Labeling – Data as the key
Some tasks are ripe for pre-annotation. For example, if you use the example from our experiment, you could use pre-annotation to label images, and a team of data labelers can determine whether to resize or delete the labels, or bounding boxes.
This reduction of labeling time can be helpful for a team that needs to annotate images at pixel-level segmentation.
Our takeaway from the experiments is that applying auto labeling requires creativity. We find that our clients who use it successfully are willing to experiment, fail, and pivot their process as necessary.
As auto data labeling is one of the breakthroughs for a better outlook of the AI technology, specifically machine learning, we still have a lot to discover with this new term.
If you want to hear from our experts concerning the matter of Automated data labeling, please contact us for further details.
- Website: https://www.lotus-qa.com/
- Tel: (+84) 24-6660-7474
- Fanpage: https://www.facebook.com/LotusQualityAssurance